Efficient Bayesian A/B experiments involve the following steps:
Posted: Wed Dec 18, 2024 10:44 am
One common option is to go with a non-informative prior, like a uniform or Jeffreys prior. These priors give equal chances to all possible outcomes. Another choice is to use informative priors based on past data or expert knowledge.
When selecting priors, it’s often a good idea to go philippines girls telegram for weaker priors than what historical data suggests. A strong prior can help guard against false discoveries, but it might require more data to spot winning variations when they’re there.
A weak prior means the data you’ve collected is given more weight. However, this can sometimes lead to false discoveries if you stop the test early.
2. Setting up experiments
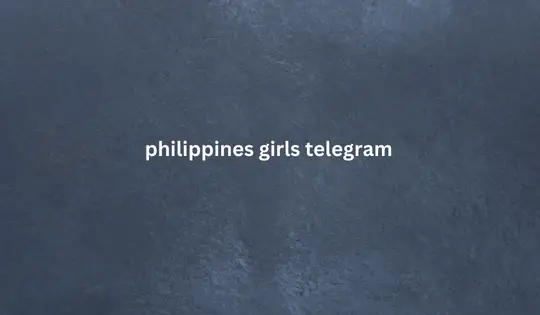
Determine the prior distribution based on the chosen metric.
Gather data for each variant and analyze it for key performance indicators (KPIs).
Employ Bayesian analysis to revise the prior distribution with the observed data.
Compare the variants using the updated posterior distributions.
For really accurate results, consider things like the expected effect size, significance level, power, and the conversion rate you typically have when deciding how big your Bayesian A/B test should be.
With careful planning and by following these best practices, you can get the most out of your Bayesian A/B testing and achieve the best results.
3. Interpreting results
Understanding the results of a Bayesian A/B test involves looking at the probability distributions of the conversion rates for each group in the experiment.
When selecting priors, it’s often a good idea to go philippines girls telegram for weaker priors than what historical data suggests. A strong prior can help guard against false discoveries, but it might require more data to spot winning variations when they’re there.
A weak prior means the data you’ve collected is given more weight. However, this can sometimes lead to false discoveries if you stop the test early.
2. Setting up experiments
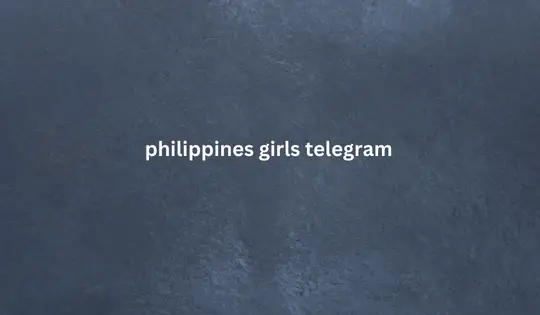
Determine the prior distribution based on the chosen metric.
Gather data for each variant and analyze it for key performance indicators (KPIs).
Employ Bayesian analysis to revise the prior distribution with the observed data.
Compare the variants using the updated posterior distributions.
For really accurate results, consider things like the expected effect size, significance level, power, and the conversion rate you typically have when deciding how big your Bayesian A/B test should be.
With careful planning and by following these best practices, you can get the most out of your Bayesian A/B testing and achieve the best results.
3. Interpreting results
Understanding the results of a Bayesian A/B test involves looking at the probability distributions of the conversion rates for each group in the experiment.